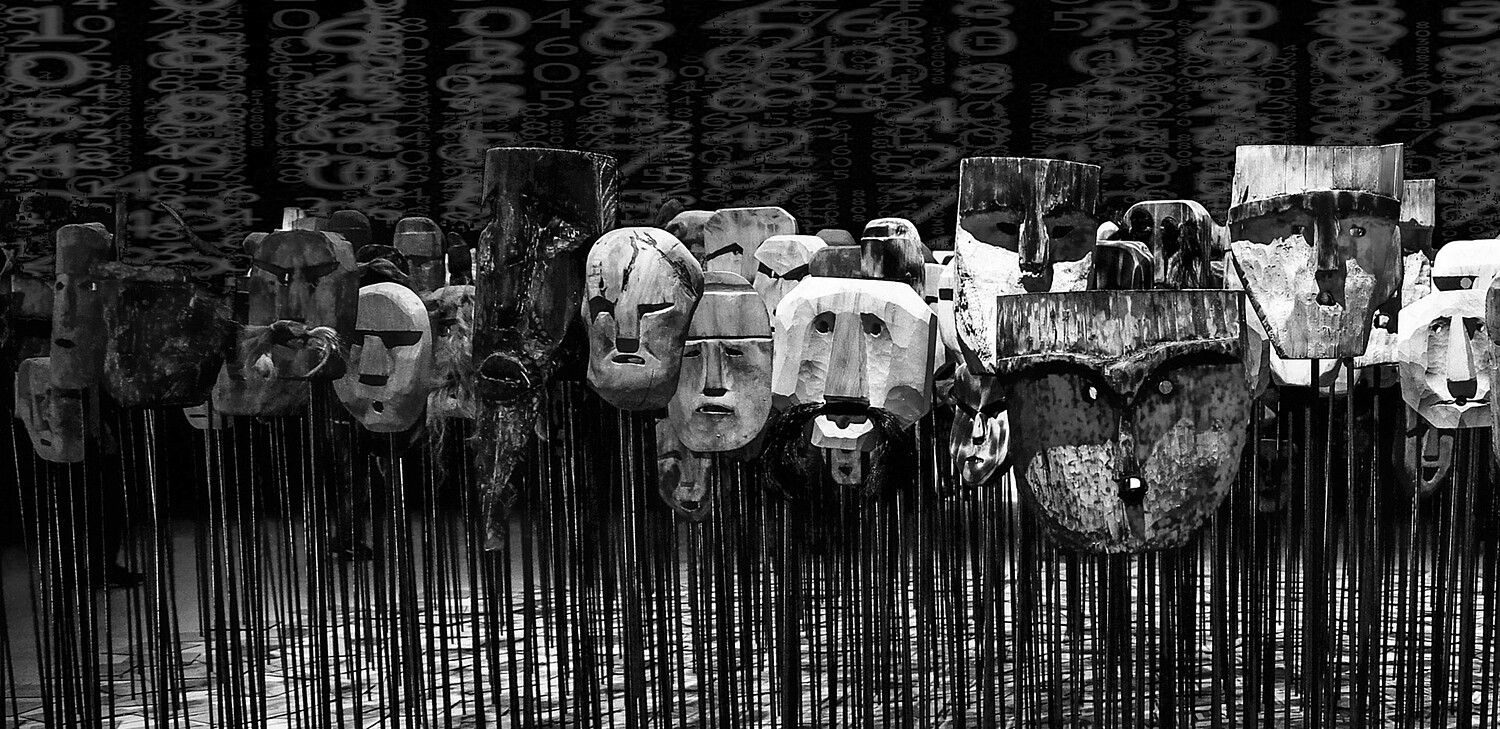
Putting a stop to lies, hatred and misleading information through artificial intelligence
The Centre for Artificial Intelligence at ZHAW is conducting research into tools for automatically detecting problematic content on the internet. These tools are aimed at helping platforms decide whether a post should be deleted. The latest project involves the development of a prediction tool for hate speech. This is to act as an early warning system to stop hatred being spread on the internet.
Some lies are short-lived. On day 50 after Russia invaded Ukraine, the Russian state news agency Tass reported the loss of the “Moskva” missile cruiser. The flagship of the Russian Black Sea Fleet had sunk in a “heavy storm”, Tass reported. Just a few hours later, weatherman Jörg Kachelmann tweeted: “The ‘Moskva’ certainly didn’t sink in a storm, because there was no storm.” Beneath his post, he included a link to the weather map for the time in question. And, indeed, all that was to be seen was a mild breeze off the coast. That served to back up what the Ukrainian armed forces had been saying. They maintained that they had sunk the ‘Moskva’ with anti-ship missiles.
Research into the detection of fake news
In many cases, however, misinformation remains undisputed for a longer period of time. “In these cases, the facts cannot be verified as readily as the weather off Odessa”, says Pius von Däniken of the Centre for Artificial Intelligence who is conducting research into methods for detecting problematic content on social media. Anything that can be rated as propaganda, fake news or conspiracy theories is suspicious. And this also includes toxic discourse such as hate speech, racism and harassment.
When profiles are abused
Various approaches can be employed to detect content of this type. Key information can be obtained by looking at the origin, for example. Profiles are repeatedly stolen and misused to spread problematic content, says von Däniken. A look at the so-called metadata will often suffice to show that this is the case: how often is someone posting? When precisely? What contact do they have with other profiles? “One thing that stands out, for example, is any change in the time of day at which posts are published”, the 31-year-old explains. Has the activity suddenly switched to the working hours for the time zone in China or Moscow? If posts are published online precisely every 15 minutes, this would indicate automated posting. Or perhaps a profile suddenly starts distributing posts from other users on a massive scale that it has never shared before.
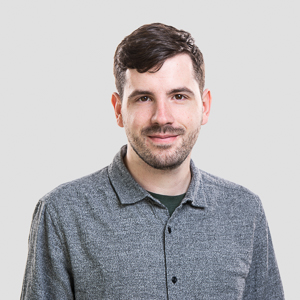
“An automatic block would be dangerous, which is why decisions must always be taken by real people.”
The content itself naturally also provides clues, such as if swear words or insulting designations point to hate speech. The big challenge, however, is the enormous quantity of posts. This is why Natural Language Processing is employed for text analyses of this type – a method positioned at the interface between linguistics and computer science. This constitutes a key focus of research at the Centre for Artificial Intelligence.
Like a spam filter, just more complex
With this method, natural language is processed algorithmically. “We have a toolbox of methods that we use for solving a range of problems”, Pius von Däniken explains. In the case of social media profiles, for example, it is frequently the classification of distributed content that is required. Can the tweet or the posted document pass for harmless? Or is it a problematic text? This can essentially be likened to the filter that decides whether an incoming mail is spam or not, says Pius von Däniken– “although it is slightly more complex than that”.
On behalf of Armasuisse
The Confederation is also interested in having fake news and hate speech detected by artificial intelligence. The Cyber Defence Campus of the Federal Office for Defence Procurement (Armasuisse) is driving research in this area forward. Cyber threats have become considerably more significant and complex and “have an increasingly critical impact on the security of our society”, says Vincent Lenders, Head of the Campus, in the Annual Report.
In an initial phase, the ZHAW team specialising in Natural Language Processing at the Centre for Artificial Intelligence updated Armasuisse on the latest developments in the different detection methods in its research project “Detection of Suspicious Social Media Activities”. The second phase now involves the development of a prediction tool for hate speech based on artificial intelligence. Used as an early warning system, it will provide an indication as to whether a user of the Twitter short message system is poised to spread hate messages. The idea is to stop hatred before it is spread on the internet.
AI trained with 200 Twitter profiles
The tool is being trained with a dataset of some 200 Twitter profiles. Roughly half of these have attracted frequent attention by posting clear-cut hate messages or insulting or offensive content. The content is assessed by three people. The other profiles happen to have published something suspicious at some time. “But that doesn’t necessarily mean anything”, says von Däniken explaining the classification of the second group. A case is not always clear, especially when no background information is available on the person in question. The situation is different if a Jewish woman posts a Jewish joke rather than a neo-Nazi. Besides, the three people conducting the assessments have frequently been unable to agree whether content was offensive or not.
Indicators of change
“We then studied the entire timeline of all the profiles to identify all their behaviour on Twitter to date”, says von Däniken. This includes not only the messages sent so far but also the network built up around an individual profile, showing the influence exerted by others. The indicators of change also include what the user in question currently reads.
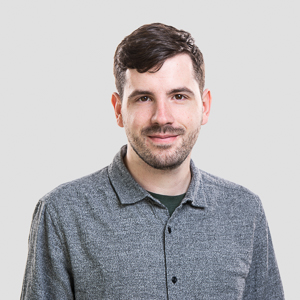
“Our aim is to model the influences that lead to this change in behaviour so that preventive action can be taken.”
It is not the clear-cut cases that have been posting hate-filled messages right from the start that are interesting. Much more exciting is a second group – the potential suspects. They perhaps got angry in a certain situation and then posted something problematic. In many cases, nothing further happened. Others, however, start to become radicalised. They get caught up in a genuine spiral of hate and then regularly spread hate messages.
“Our aim is to model the influences that lead to this change in behaviour”, the researcher explains. The corresponding profiles are then put under observation. And if a threat of hate messages arises, the prediction tool will issue an alarm so that they can be prevented from spreading before it’s too late. Another option would be to inform the person that they were now crossing the line of what is permissible.
Pre-selection by the tool
Should the account be automatically blocked? Pius von Däniken does not think that would be right. There is always an ethical component to censorship, he says, and there is a danger that freedom of expression could be unjustifiably curtailed. An automated reaction would be dangerous; the decision must always be taken by real people.” The researcher also regards this approach as being significantly more efficient. A tool of this kind would have advantages if it pre-selected suspicious content and left it up to humans to take the decisions. Machines are very good at recognising suspicious patterns. They can very rapidly sift through the enormous quantities of data that social media generate on the internet every hour.
Acceptable jokes or hate speech?
“When it comes to decision-making, however, humans are more efficient”, stresses von Däniken. Humans can quickly work through problem cases arranged hierarchically according to the level of suspicion. People are in a better position to distinguish between acceptable jokes and hate speech. Or between offensive and harmless content. This is not the case for the Facebook algorithm, which has already made a fool of itself several times in the past by censoring the “Venus of Willendorf” stone-age statue for allegedly being offensive along with many other established works of art.
The same applies when it comes to obtaining the decisive facts for verifying the truth content of a questionable message. Humans are more adept at recognising the relevant points in a given situation and at selectively comparing these with the correct, reliable source and hence, as in the case with the “Moskva”, distinguishing stormy weather from calm weather and lies from the truth.
How frequently are young people in Switzerland exposed to online hatred and fake news?
The Media Psychology Section at ZHAW publishes the so-called JAMES Study at regular intervals showing how young people use the media in Switzerland. On the basis of the 2020 study, the Centre for Artificial Intelligence is now looking into how frequently hate messages circulate in the relevant networks. The research project is also setting out to characterise the actors behind these messages. It adopts two approaches here. “On the one hand, we focus on data from Jodel.ch, a platform that young people use to communicate locally”, project leader Pius von Däniken explains. If someone posts a message online, only those within a radius of a few kilometres will see it. And, on the other hand, we analyse tweets from 2021 relating to polarising referendums, such as on the burqa ban, marriage for everyone and the Covid-19 law. The project is currently in the evaluation phase.
0 Comments
Be the First to Comment!